SOPHIA ANTIPOLIS, France – February 24, 2023 │ KnowMade releases a new report to investigate the patents related to memory technologies for artificial intelligence/machine learning (AI/ML) applications.
Creating artificial neurons and synapses using emerging memories
In recent years, neuromorphic computing has emerged as a promising technology in the post-Moore’s law era. Neuromorphic computing systems are highly connected and parallel and consume relatively low power and processes in memory. Artificial neurons and synapses that mimic biological ones are needed to implement such a system on hardware. Both must be power-efficient, scalable, and capable of implementing relevant learning rules to facilitate large-scale neuromorphic functions. To this end, numerous efforts have been made over the last few years to create artificial neurons and synapses using emerging memories, including magnetoresistive random-access memory (MRAM), phase-change memory (PCM), resistive random-access memory (ReRAM or memristors), conductive bridging random-access memory (CB-RAM), ferroelectric random-access memory (FeRAM), ferroelectric field-effect transistor (FeFET), synaptic transistors, and others.
In this context, KnowMade is releasing a new report that aims to provide a comprehensive view of the patent landscape related to memory technologies for artificial intelligence/machine learning (AI/ML) applications, from materials and devices to the systems and methods that use them, categorized into memory technologies (ReRAM, PCM, MRAM, FRAM/FeFET, Flash, DRAM, SRAM, etc.).
In this report, we aim to answer the following questions:
- Is there a specific memory technology more suitable than others for artificial intelligence/machine learning (AI/ML)?
- Who are the main patent owners and patent applicants in the field?
- What are the technical challenges targeted in patents, and what is protected (device, system, method, etc.)?
- Are there memory properties developed more specifically for intelligence/machine learning (AI/ML)?
Why studying the patent landscape
Patent landscape analysis is a powerful tool for understanding the competitive and technological environment. It makes it possible to identify new players in emerging industries long before they enter the market while providing a better understanding of their expertise and know-how of a specific technology. Overall, patenting activity (patent filings) reflects the level of R&D investment made by a country or player in a specific technology while providing clues as to the technology readiness level reached by the main IP players. What’s more, the technology coverage and the geographical coverage of the patent portfolios are closely related to the business strategy of IP players.
A diversified ecosystem of semiconductor companies
A mix of IC and memory players, universities, and R&D centers are competing for innovation at all stages of the R&D ladder. Industrial companies’ patenting activity took off in 2015 after R&D efforts were focused on research and fundamental physics knowledge. American and Korean industrials and Chinese and Korean universities mainly hold patents. Top patent assignees are well-established semiconductor companies, and IBM and Samsung have a leading IP position. New players such as Applied Materials, TSMC, GlobalFoundries, TetraMem, and ICLeague are entering the game, and their intellectual property (IP) may become important in the coming years.
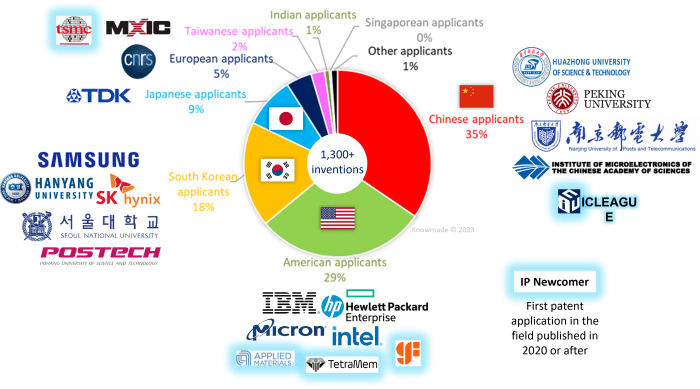
Figure 1: Geographical distribution of patent applicants for memory technologies targeting artificial intelligence/machine learning applications.
All emerging memories are currently under investigation
The patents have been categorized according to the memory technologies developed for neuromorphic computation: resistance-based emerging memory technology (ReRAM, PCM, MRAM), polarization-based emerging memory technology (FRAM/FeFET), traditional memory technologies (Flash, DRAM, SRAM), other memory technologies (synaptic ionic transistor, devices based on 2D or 1D materials, hybrid materials, perovskites, nanoparticles, quantum physics, organic materials, skyrmions, etc.), and not specific (type of memory technology not specified).
All emerging memories are currently under investigation, with an upward trend for protecting FRAM in recent years. RRAM is the technology with the most inventions and the most significant number of enforceable patents worldwide.
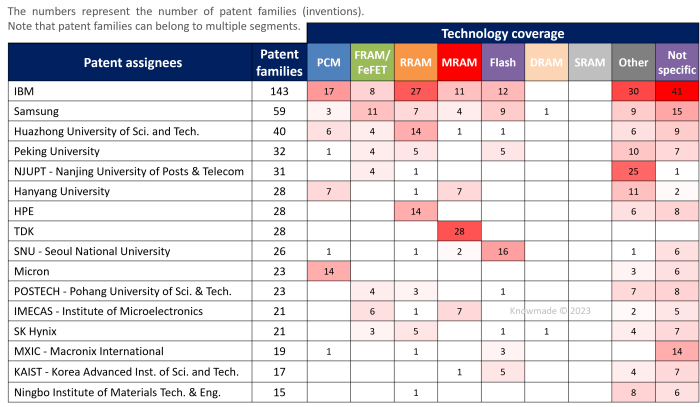
Figure 2: Technology coverage of main patent assignees’ IP portfolio related to memory technologies targeting artificial intelligence/machine learning applications.
In this report, the IP portfolios of eight key players have been deeper investigated: IBM, Samsung, Applied Materials, TDK, SK hynix, Macronix, HP, and TetraMem. Each player’s patent portfolio related to memory technologies for AI/ML applications is analyzed to provide an overview of its strengths, potential for reinforcement, level of IP activity, main IP collaborations, recent patenting activity, and inventions that stand out. Furthermore, this report includes an extensive Excel database with the 1,300+ patent families (inventions) analyzed in this study. This useful patent database allows for multicriteria searches and includes patent publication numbers, hyperlinks to an updated online database (original documents, legal status, etc.), priority date, title, abstract, patent assignees, patent’s current legal status, and nine segments (RRAM, PCM, MRAM, FeRAM/FeFET, Flash, DRAM, SRAM, other, not specific).
Our experts have conducted additional studies in this field; explore our patent landscapes on memory.
Press contact
contact@knowmade.fr
Le Drakkar, 2405 route des Dolines, 06560 Valbonne Sophia Antipolis, France
www.knowmade.com
About the author
Pauline Calka, PhD. works for KnowMade as a patent analyst in the fields of semiconductor manufacturing and advanced packaging. She holds a PhD in Memory from the University of Grenoble Alpes (France), in partnership with the CEA-Leti (France). After an Alexander von Humboldt Postdoc fellowship position at the Technical University of Berlin (Germany) and the Leibniz Institute for High Performance Microelectronics on ReRAM development, Pauline worked five years at ASM International (Belgium) as Senior Process Engineer on thin film development for logic, memory and MEMS, and two years at CEA-Leti as Integration Engineer on imaging CMOS sensors.
About KnowMade
KnowMade is a technology intelligence and IP strategy consulting company specialized in analyzing patents and scientific publications. The company helps innovative companies, investors, and R&D organizations to understand competitive landscape, follow technological evolutions, reduce uncertainties, and identify opportunities and risks in terms of technology and intellectual property.
KnowMade’s analysts combine their strong technology expertise and in-depth knowledge of patents with powerful analytics tools and methodologies to turn patent information and scientific literature into actionable insights, providing high added value reports for decision makers working in R&D, innovation strategy, intellectual property, and marketing. Our experts provide prior art search, patent landscape analysis, freedom-to-operate analysis, IP due diligence, and monitoring services.
KnowMade has a solid expertise in Compound Semiconductors, Power Electronics, Batteries, RF Technologies & Wireless Communications, Solid-State Lighting & Display, Photonics, Memories, MEMS & Sensors, Semiconductor Packaging, Medical Devices, Medical Imaging, Microfluidics, Biotechnology, Pharmaceutics, and Agri-Food.